Inference Scaling for Long-Context RAG
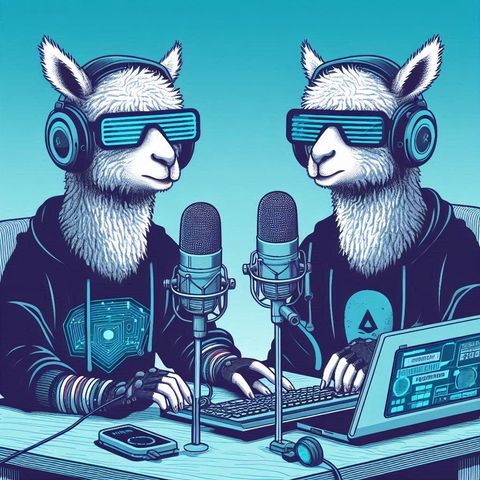
Download and listen anywhere
Download your favorite episodes and enjoy them, wherever you are! Sign up or log in now to access offline listening.
Description
🗓 Inference Scaling for Long-Context Retrieval Augmented Generation This research paper explores the effectiveness of inference scaling for retrieval augmented generation (RAG), a technique that enhances large language models (LLMs)...
show moreThis research paper explores the effectiveness of inference scaling for retrieval augmented generation (RAG), a technique that enhances large language models (LLMs) by incorporating external knowledge. The authors introduce two strategies, demonstration-based RAG (DRAG) and iterative demonstration-based RAG (IterDRAG), for effectively scaling inference computation. They demonstrate that increasing inference computation, when optimally allocated, leads to nearly linear gains in RAG performance. Furthermore, they develop a computation allocation model to predict the optimal test-time compute allocation for various tasks and scenarios, showcasing its effectiveness in achieving performance gains and aligning with experimental results.
📎 Link to paper
Information
Author | Shahriar Shariati |
Organization | Shahriar Shariati |
Website | - |
Tags |
Copyright 2024 - Spreaker Inc. an iHeartMedia Company
Comments